Radiant MLHub Spotlight Q&A: Mohammad Alasawdah
Meet Mohammad Alasawdah, our Community Voice for the last quarter of 2022. Mohammad is an Earth observation and climate data science researcher at Eurac Research, focusing on issues that affect people, their health, and the environment around them. The organization aims to improve life in the societies of the future.
Mohammad holds a joint master of science degree in geospatial technologies from the University of Münster, NOVA University, and Jaume I University. His research projects include using python to create an artificial neural network to classify land cover. Other work has included examining the relationship between seismic data and potential damage by compiling landslide risk mapping and analyzing change detection for emergency management using Google Earth Engine.
Earlier this year, Mohammad joined forces with Emmanuel Siaw-Darko to win third place in the AI4FoodSecurity data challenge for their model to classify crop types in South Africa and Germany. Emmanuel, who accepted a machine learning internship with Radiant Earth as an award for winning the competition, credits Mohammad for helping him prepare satellite imagery and render data to extract insights from it. This includes learning how to match the labels assigned to satellite imagery to confirm the details as input data that an algorithm can use to determine patterns.
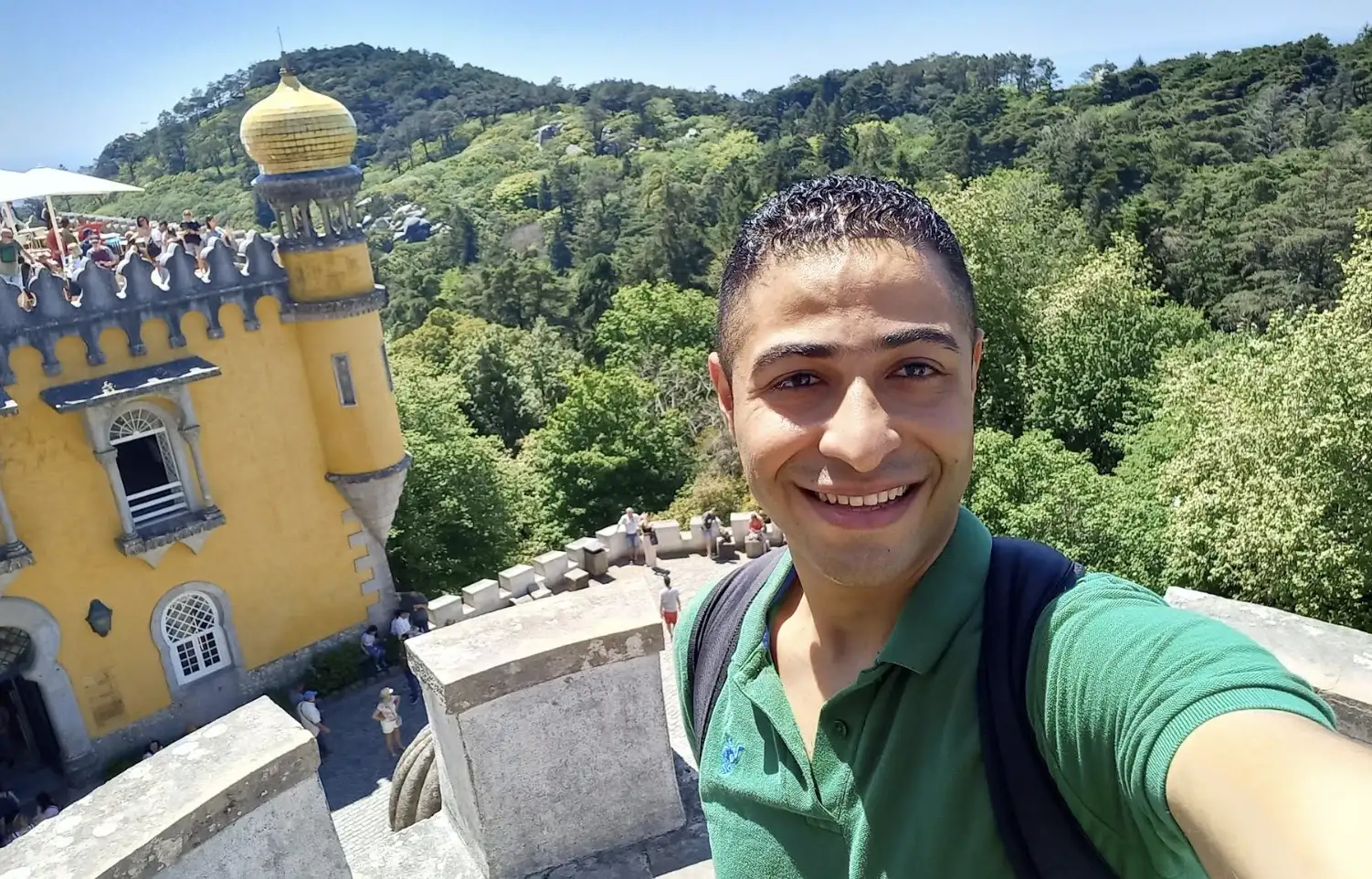
Meet Mohammad Alasawdah
In this Q&A, Mohammad talks to us about developing climate change related models and the importance of finding high-quality machine learning ready data sources.
“Simply speaking, this kind of open library, [Radiant MLHub], makes our life as researchers easier. Numerous research projects have ended, and many researchers have stopped their experiments or given up testing ideas due to the lack of datasets.”
You are skilled in spatial analysis and various programming languages and tools, and are interested in climate change and Earth science modeling. What inspired you to pursue this field? Tell us about your machine learning journey.
After graduating with my bachelor’s, I worked as a GIS specialist focusing on natural disasters and climate change effects. This work raised my awareness of the importance of finding solutions to mitigate risk. I’ve spent around 4 years working in this field, creating risk and vulnerability maps and designing spatial data infrastructure databases. During this period, I kept asking myself how we could prevent or predict risks arising from climate disasters and better prepare ourselves for catastrophes. I didn’t know about AI/ML and how we could use them at that time. Struck with the monotonous workflow of designing geospatial maps and implementing systems and databases, I began to look for a new opportunity to improve my skills and find a new challenge. Thanks to a scholarship funded by the European Commission, I pursued a Master of Science in Geospatial Technologies in Germany, Portugal, and Spain.
This joint master’s was excellent; I took different courses in geostatistics, geospatial data mining, and artificial intelligence. I’ve learned how to use these new techniques to solve real environmental problems by doing many projects during my study with monitoring from experts and professors in the field. After graduation, I was still hungry to apply what I’d learned. To stay close to the field and maintain my skills, I started participating in different challenges to solve real-world problems. Winning some of them — such as taking 2nd place in the HYPERVIEW challenge organized by the European Space Agency — was very rewarding. I was making a difference in this world using my skills.
There are many challenges with building ML applications using Earth observation data, such as (lack of) diversity and bias in data and the ability to scale research applications to real-world solutions. What challenges have you found most problematic?
One of the biggest challenges is computing resources. I’ve often been requested to build a solution using big Earth observation data, but that requires massive computing resources to build a robust model or even to process the EO data before starting to build AI/ML applications. Research centers are struggling with computing source limitations and sometimes have to wait days to see their results. I have memories of preprocessing Sentinel-2 data and generating different indices over a large area; Storing them in a database would take days to complete. This makes it more challenging to scale the developed applications to real-world solutions.
Another anecdote about computing resources comes from when I competed against a German computing center to predict soil parameters using hyperspectral data. Using my available resources, I had to wait hours to see the results of a simple random forest model while it took the computing center around 5 minutes. I couldn’t build any CNN/RNN models, and they produced them quickly. I wonder about the transferability of such a solution to the real world and making it available worldwide.
“…it’s incredible that Radiant MLHub offers free access to very well-structured datasets available for immediate work without complicated processing or analyzing. What I really like about [it] is the diversity in datasets, which fills the gap and improves the availability of data representative of developing countries.”
As you know, Radiant Earth has various open-access training datasets available on Radiant MLHub. What do you see as the potential of Radiant MLHub for researchers like you?
Simply speaking, this kind of open library makes our life as researchers easier. Numerous research projects have ended, and many researchers have stopped their experiments or given up testing ideas due to the lack of datasets. Or researchers do not have enough funds to generate data or get access to such data. So, it’s incredible that Radiant MLHub offers free access to very well-structured datasets available for immediate work without complicated processing or analyzing. What I really like about Radiant MLHub is the diversity in datasets, which fills the gap and improves the availability of data representative of developing countries. When planning for my master’s thesis to analyze crop diseases in Africa to support food security, I had to change my topic because I couldn’t find suitable data sources for Africa. I think young researchers will be more than happy with Radiant MLHub.
Which specific training datasets on Radiant MLHub have you used, and for what purpose(s)?
I’ve used flood datasets like the “NASA Flood Extent Detection.” But I did not use them to build an AI/ML application. Instead, I used the datasets to get insight into what data for flood detection looks like. I then applied my observation to generate a similar dataset to help detect excess water in Latvia. This work was done in collaboration with FruitPunch AI, SUN — Space Hub Network, the geospatial analytics company Baltic Satellite Service, and Forest Radar, which use machine learning and satellite imagery for advanced forest intelligence.
I have also used various crop type datasets like the “A Fusion Dataset for Crop Type Classification in Germany.” I’ve used these datasets in different challenges focusing on food security and land management by extracting useful information to classify crops in the same season, and take a step further by exploiting the time dimension in time series data to extrapolate classification into different growing seasons.
As a follow-up to the previous question: Can you share insights on how the model(s) performed or implemented?
Detecting flooded areas in the forest is painful, but we got a good result that can be built upon for the future and give better insight to direct future research. Different models have been developed and tested, from tree-based models to more advanced techniques like U-Net and inception networks. Our results proved that it’s possible to use coarse-resolution satellite images to detect flooded areas by achieving a 74% accuracy rate using the inception-v4 model and a 71% IOU using U-Net. The results are promising, considering we used a few bands (4 bands) without including other important indices like NDVI and NDWI.
For the crop type datasets, I focused on balancing the accuracy of classification and computing resources for good performance without needing outlandish resources. I came up with an idea to restructure the data into tabular data. I then used a mix of light tree-based models and applied some weighting techniques to classify the crops with the ability to have more resources available to improve the classification by tuning the models and adding more features.
What is your hope for scalable machine learning on satellite data related to sustainability projects like climate change? What do you need to scale what you have built so far?
More collaboration between AI & EO experts would be great and helpful in building robust and sufficient solutions that can be generalized very well. This kind of collaboration would guarantee reasonable exploitation of the latest cutting-edge techniques on both sides.
ML and data ethics for Earth observations applications are important to scale the research solutions to real-world applications. I hope the AI4EO community can have clear ethics outlines/principles and put them into practice. In this way, we could guarantee a good solution and avoid manipulating the data or the ML model to present biased results just to get an instant victory. Also, clear ethics would really encourage more people to share their data/code because they know that their data will be safe and used in a meaningful way.
We also need more advanced computing platforms to support building training datasets for more developed AI/ML models using geospatial data. We have Google Earth Engine (GEE), the most known platform, but it is still limited. Platforms like GEE enable access to various spatial data; however, it still does not allow for building complex ML/AI models. The ability to build AI/ML models and then compute them on the back end will be helpful for researchers to create diverse solutions to help people in different aspects of their life.
From your perspective, what ML and spatial analysis innovations can we expect in the next decade, and how do you think they might improve people’s lives?
I expect to have more smartphone applications used by people in their daily life. The importance of such apps was raised during the COVID-19 pandemic; for example, apps to warn you when you’re in a risk zone or show you the vaccination distribution. But this also takes us back to the importance of data ethics to let people trust and share their data. Maybe another app could help if you want to buy a farm to show you a quick overview of the soil properties and the history of crops planted on the farm. Actually, I could not stop myself from thinking about innovative ideas that could be applied in the future using ML and spatial analysis, so let’s build our models and enjoy what is coming 😊.